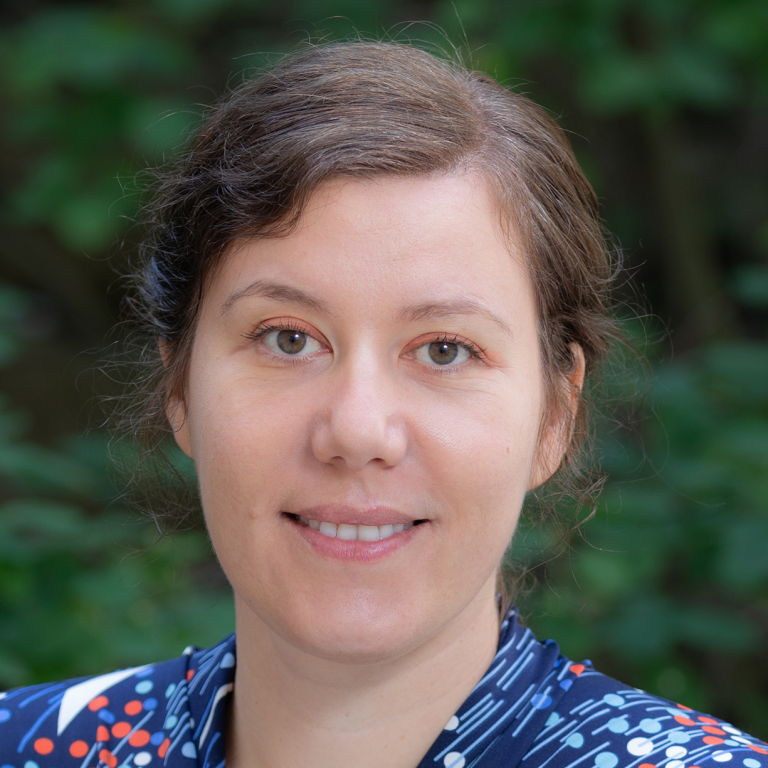
Larissa Albantakis, PhD
Assistant Professor of Psychiatry
Larissa Albantakis is a computational neuroscientist and Assistant Professor at the UW-Madison Department of Psychiatry. Her research explores the relationship between causation, complexity, consciousness, and cognition, and their quantitative assessments in neural network models and neurophysiological data from healthy subjects and clinical patient populations.
Dr. Albantakis obtained her Diploma (MSc) in Physics from Ludwig-Maximilians University in Munich in 2007, and her PhD in Computational Neuroscience from Universitat Pompeu Fabra in Barcelona in 2011 under the supervision of Gustavo Deco. Her PhD research focussed on using large-scale, biophysically-realistic neural models and dynamical systems theory to understand the neural mechanisms underlying sensorimotor decision-making in contexts where subjects have multiple choice-alternatives and the option to “change one’s mind.”
Dr. Albantakis has been at the University of Wisconsin-Madison since 2012, where she worked together with Dr. Giulio Tononi before starting her own research group in 2022. Her work with Dr. Tononi proved essential for developing (1) the mathematical formalism of the integrated information theory (IIT) of consciousness; (2) a demonstration of the adaptive advantages of recurrent architectures and high information integration in evolving artificial organisms; (3) a body of work assessing causal relations across micro and macro spatio-temporal scales in artificial neural networks; (4) an account of the relation between causal and dynamical complexity in discrete dynamical systems; (5) a quantitative framework of actual causation (“what caused what”); and (5) a formal account of informational autonomy of an agent from its environment (see publication record below).
Her blog “Conscious(ness) Realist” provides publication reviews and commentaries on topics related to consciousness science and its theories.
Research Interests: Computational Psychiatry, Schizophrenia, Causation and Causal Analysis, Consciousness, Cognition, Information, Complex System Science, (Artificial) Neural Networks, Decision-making, Free Will, Artificial Life/Intelligence.
Albantakis L (2021) Quantifying the Autonomy of Structurally Diverse Automata: A Comparison of Candidate Measures. Entropy, 23(11): 1415. doi: 10.3390/e23111415
Grasso* M, Albantakis* L, Lang JP, Tononi G (2021) Causal reductionism and causal structures. Nature Neuroscience, 24: 1348-1355. doi: 10.1038/s41593-021-00911-8
Albantakis L, Marshall W, Hoel E, Tononi G (2019) What Caused What? A Quantitative Account of Actual Causation Using Dynamical Causal Networks. Entropy, 21:459. doi: 10.3390/e21050459
Albantakis L, Tononi G (2019) Causal Composition: Structural Differences among Dynamically Equivalent Systems. Entropy, 2019, Vol 21, Page 989 21:989. doi: 10.3390/e21100989
Marshall W, Albantakis L, Tononi G (2018) Black-boxing and cause-effect power. PLOS Comput Biol, 14:e1006114. doi: 10.1371/journal.pcbi.1006114
Marshall W, Kim H, Walker SI, Tononi G, Albantakis L (2017) How causal analysis can reveal autonomy in models of biological systems. Philos Trans A Math Phys Eng Sci 375:20160358. doi: 10.1098/rsta.2016.0358
Hoel EP, Albantakis L, Marshall W, Tononi G (2016) Can the macro beat the micro? Integrated information across spatiotemporal scales. Neurosci Conscious, 2016. doi: 10.1093/nc/niw012
Albantakis L, Tononi G (2015) The Intrinsic Cause-Effect Power of Discrete Dynamical Systems—From Elementary Cellular Automata to Adapting Animats. Entropy, 17:5472–5502. doi: 10.3390/e17085472
Albantakis L, Hintze A, Koch C, Adami C, Tononi G (2014) Evolution of Integrated Causal Structures in Animats Exposed to Environments of Increasing Complexity. PLoS Comput Biol, 10:e1003966. doi: 10.1371/journal.pcbi.1003966
Oizumi* M, Albantakis* L, Tononi G (2014) From the Phenomenology to the Mechanisms of Consciousness: Integrated Information Theory 3.0. PLoS Comput Biol, 10:e1003588. doi: 10.1371/journal.pcbi.1003588
Hoel EP, Albantakis L, Tononi G (2013) Quantifying causal emergence shows that macro can beat micro. PNAS, 110:19790–19795. doi: 10.1073/pnas.1314922110